
- This event has passed.
Thesis Defence: A Machine Learning Approach to Computed Tomography Organ Segmentation for Quality Assurance
August 14, 2024 at 9:00 am - 1:00 pm
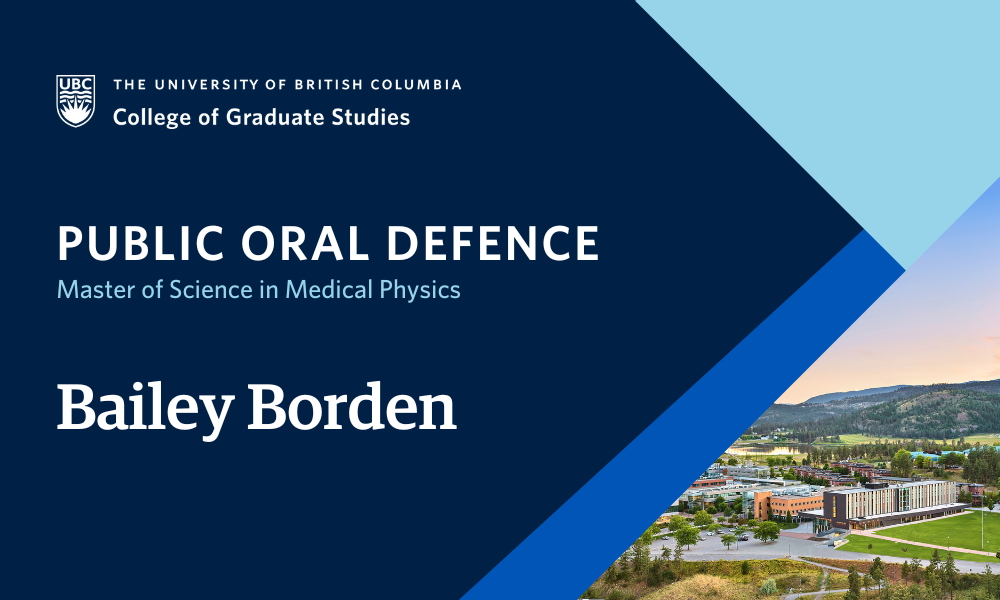
Bailey Borden, supervised by Dr. Rebecca Feldman and Dr. Rasika Rajapakshe, will defend their thesis titled “A Machine Learning Approach to Computed Tomography Organ Segmentation for Quality Assurance” in partial fulfillment of the requirements for the degree of Master of Science in Medical Physics.
An abstract for Bailey Borden’s thesis is included below.
Defences are open to all members of the campus community as well as the general public. Registration is not required for in-person defences.
ABSTRACT
Purpose: With the recent advances in computer vision, artificial intelligence (AI) autocontouring tools are becoming readily available, and can also be created using published deep neural network (DNN) frameworks. With the labour demand required to peer review cases, contouring peer review may not occur prior to the beginning of treatment. This work investigates the use of of AI for autocontouring to aid within the breast radiotherapy contouring peer review process, with the goal of helping with labour issues, and to allow patient cases to be reviewed earlier in treatment.
Methods: Using open source deep learning libraries, DNNs were trained to segment the heart, the left lung, and the right lung. The model architectures explored in this work are U-Net and LinkNet. Once training was complete, the trained U-Net model architecture was selected to demonstrate uncertainty quantification within machine learning models using conformal prediction. Additionally, a commercial network was also used to demonstrate an approach to understanding model uncertainty using test time augmentation (TTA). Furthermore, suggestions are made on selecting appropriate metrics to use within this quality assurance (QA) process.
Results: For multiclass segmentation explored, U-net and Linknet achieved a mean intersection over union (IoU) of 0.924 and 0.910 respectively. When the trained algorithms were compared to the commercial algorithm, U-Net and LinkNet achieved mean IoUs of 0.914 and 0.911, while the commercial algorithm achieved a mean IoU of 0.911. Using the conformal prediction methodology on U-Net, uncertain pixels within the model’s predictions were found to be on the boundaries of structures. When testing the uncertainty of the commercial algorithm using TTA, a standard error of 4.95% was observed on the heart predictions, 3.54% on the left lung predictions, and 3.35% on the right lung predictions.
Conclusion: For QA purposes, evaluating contours using both area and distance-based metrics is recommended. Testing of a selected model using a sample of data representing the potential patient demographic is recommended. Additionally, testing the uncertainty of a selected model using unseen data is recommended, to provide model insight. The appropriate use of these AI algorithms could aid in the contouring peer review process.