
- This event has passed.
Thesis Defence: Classifying riparian forests of the Okanagan Basin, British Columbia, Canada through random forest modelling with LiDAR and spectral derived parameters
September 6 at 12:30 pm - 1:30 pm
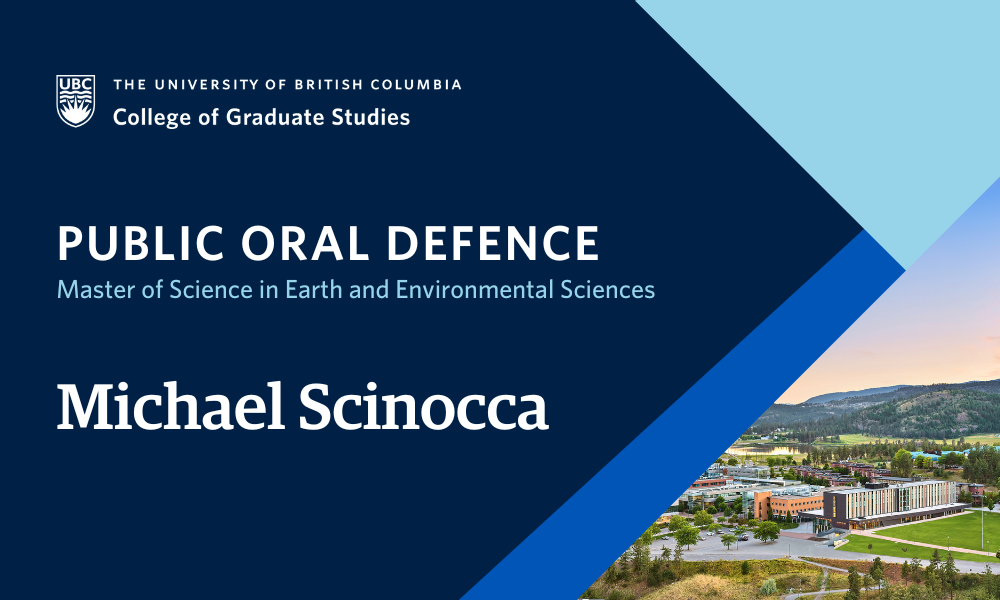
Michael Scinocca, supervised by Dr. Mathieu Bourbonnais, will defend their thesis titled “Classifying riparian forests of the Okanagan Basin, British Columbia, Canada through random forest modelling with LiDAR and spectral derived parameters” in partial fulfillment of the requirements for the degree of Master of Science in Earth and Environmental Sciences.
An abstract for Michael Scinocca’s thesis is included below.
Defences are open to all members of the campus community as well as the general public. Please email mathieu.bourbonnais@ubc.ca to receive the Zoom link for this defence.
ABSTRACT
Riparian forests are integral components of landscapes due to their ecological significance and the services they provide to both terrestrial and aquatic ecosystems. These unique ecosystems act as buffers, regulating water quality, stabilizing streambanks, providing habitat for diverse flora and fauna, and influencing local microclimates. Accurate assessment and classification of riparian forests are essential for effective land management and conservation efforts, especially in regions like the Okanagan Basin, where water resources are particularly vulnerable to anthropogenic impacts and climate change. Recent advancements in remote sensing (RS) technologies, particularly the integration and availability of multispectral and LiDAR data, offer opportunities to predict and delineate riparian forest composition at larger scales and over potentially inaccessible terrain, enabling comprehensive assessments of these ecosystems’ spatial distribution, structural attributes, and species composition. This study integrated fine-scale RGB RS imagery with LiDAR data to classify riparian forest tree species in select fish-bearing streams within the Okanagan River system, to develop a reproducible and cost-effective process for predicting ten native tree species classes and one Infrastructure class. To inform the random forest (RF) classification model, a total of 23 explanatory covariates created from LiDAR and RGB data were incorporated. The model was trained using 1,611 observations, collected through in-situ field visits and supplemented by open-access tree inventory data, sourced from various reaches of the study area, encompassing diverse locations, elevations, and ecosystem types. The RF model achieved an overall accuracy of 96.03%, evaluated through a confusion matrix. In the 100m buffers used to describe riparia of the study area, (129,802 ha), 85,679 ha (66% of the area) were classified into 11 classes. Spruce was the most predicted class (54.16% of classified cells), followed by Western Redcedar (15.01%), and Lodgepole Pine (7.46%). Of the 23 explanatory covariates used, LiDAR-derived covariates were found to be more influential than RGB-derived covariates. This research provides valuable insights for land management strategies and conservation efforts, especially in regions like the Okanagan Basin, where water resources are particularly vulnerable to anthropogenic impacts and climate change.