
- This event has passed.
Thesis Defence: Enhanced Clustering and Classification of Alarm Floods Based on an Information-Theoretic Approach
October 11, 2024 at 9:00 am - 1:00 pm
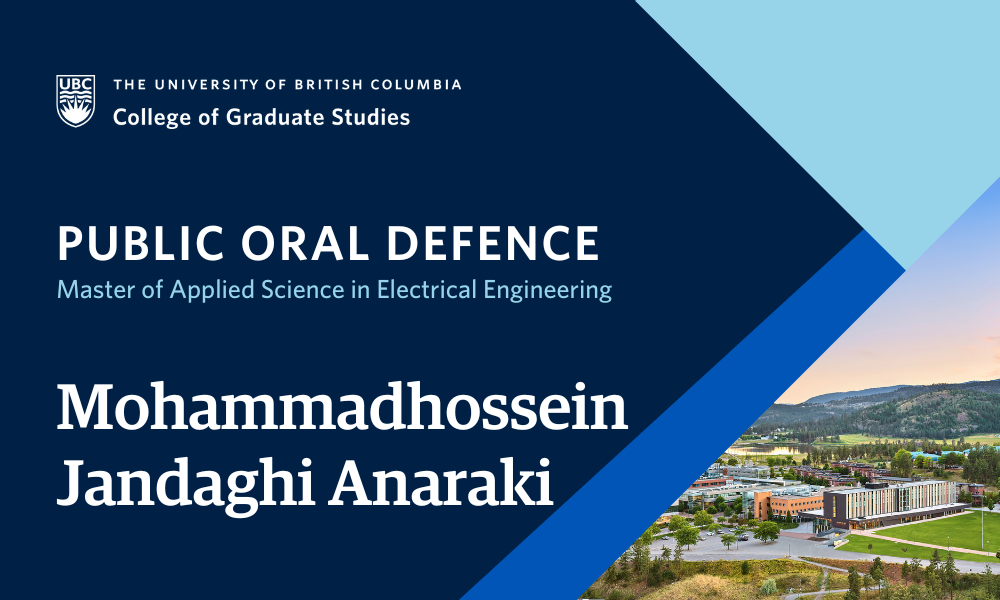
Mohammadhossein Jandaghi Anaraki, supervised by Dr. Ahmad Al-Dabbagh, will defend their thesis titled “Enhanced Clustering and Classification of Alarm Floods Based on an Information-Theoretic Approach” in partial fulfillment of the requirements for the degree of Master of Applied Science in Electrical Engineering.
An abstract for Mohammadhossein Jandaghi Anaraki’s thesis is included below.
Defences are open to all members of the campus community as well as the general public. Please email ahmad.aldabbagh@ubc.ca to receive the Zoom link for this defence.
ABSTRACT
Safety in industrial sectors has become a critical concern, ensuring the well-being of workers, the protection of assets, and the prevention of environmental damage. Effective monitoring of industrial processes is essential to maintain smooth operation and prevent potentially dangerous incidents. To achieve this, industrial control systems play a pivotal role by providing real-time monitoring and control capabilities.
Alarm systems are an integral part of industrial control systems. They alert operators about any deviations from normal operation conditions. When a fault occurs in an industrial system, an alarm is activated to alert the operator about the abnormal situation. When an alarm annunciates in a control system, a timely response by the operator can return the process to normal conditions and prevent catastrophic incidents.
However, there are some cases in which multiple alarms are annunciated at once, creating a phenomenon known as an “alarm flood.” Alarm floods can overwhelm operators responsible for monitoring and managing industrial systems, making it difficult to make quick and effective decisions. This represents one of the most significant challenges in industrial control systems.
This study presents an innovative approach for the clustering and classification of alarm floods in industrial systems, addressing the challenge of managing overwhelming alarm annunciations in complex processes. The research focuses on the development of a novel representation for alarms and alarm floods, which enables the detection and analysis of changes in alarm flood sequences over time due to varying operational conditions, environmental factors, or modifications in system settings. This study introduces dynamic clustering, which allows alarm floods clustering and classification, even in the absence of historical alarm data. This approach assists operators in handling abnormal conditions more accurately, thereby improving plant safety and efficiency.