
- This event has passed.
Dissertation Defence: Databiting: Enabling Lightweight, Transient, and In-Situ Exploration of Personal Fitness Data on Smartwatches
October 25 at 8:30 am - 12:30 pm
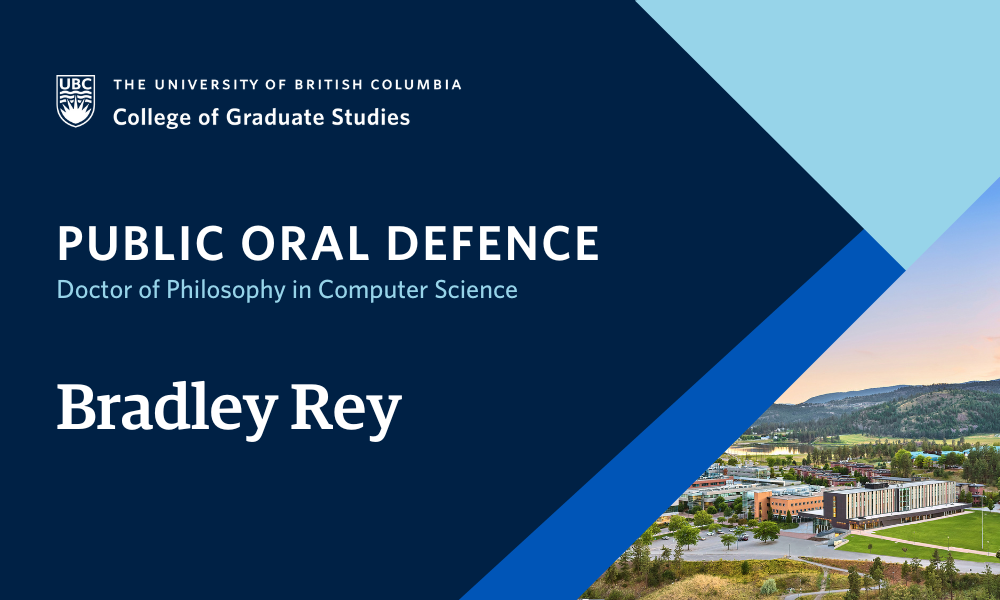
Bradley Rey, supervised by Dr. Pourang Irani, will defend their dissertation titled “Databiting: Enabling Lightweight, Transient, and In-Situ Exploration of Personal Fitness Data on Smartwatches” in partial fulfillment of the requirements for the degree of Doctor of Philosophy in Computer Science.
An abstract for Bradley Rey’s dissertation is included below.
Examinations are open to all members of the campus community as well as the general public. Registration is not required for in person exams.
ABSTRACT
Smartwatches have become increasingly popular for health and fitness tracking. Worn on the body, and equipped with powerful sensors, smartwatches enable comprehensive and ubiquitous health data collection. Exploring this collected data on the smartwatch is, however, less comprehensive and is largely unrealized. The small screen size and many daily scenarios of use are often considered challenges which limit exploratory capability. Yet, the immediate availability of the smartwatch remains to offer potential for actionable and in-situ insights derived from the collected data. This thesis introduces databiting, a novel concept for lightweight and transient data exploration that supports in-situ exploration on the smartwatch. Databiting bridges the gap between currently offered glanceable data visualizations, and more extensive data analysis available on other devices.
Before realizing databiting, we conducted an empirical study involving 18 people over one week to understand users’ smartwatch data exploration needs. We captured and analyzed 205 personal health data queries to understand where, when, and why these queries were desired. This analysis combined qualitative insights with quantitative metrics, uncovering patterns that can inform the design and implementation of databiting on the smartwatch. We further identified key dimensions of these queries, such as interrogatives, data sources, aggregations, and filtering mechanisms, which provide a foundation for enhancing natural language processing capabilities.
We then examined user preferences of voice assistant responses to personal health data queries, as an additional output modality to visualization. Insights were gathered on perceived quality, behavior, comprehensibility, and efficiency of these answer structures, providing guidelines to support databiting through an effective output modality during cognitively loaded tasks, such as during a workout.
Using these findings, we then developed DataWatch, a novel smartwatch application which facilitates in-situ exploration of past collected fitness data through lightweight and transient touch and speech interactions. DataWatch supports Single Value, Browse, and Compare exploration types. Furthermore, users can explore their data during different workout phases—before, during, and after a workout— enhancing one’s ability to gain insights and make informed decisions while in-situ.
Overall, this research demonstrates the feasibility and benefits of databiting on smartwatches, providing a foundation for future advancements in personalized data exploration on wearable devices. DataWatch exemplifies how smartwatch health applications can begin to evolve within their means to meet user expectations, enhance the user experience, provide increased actionable insights, and motivate deeper engagement with health data.