
Thesis Defence: Kolmogorov-Arnold Networks for Spectrum Sensing and Modulation Classification
October 31 at 9:00 am - 1:00 pm
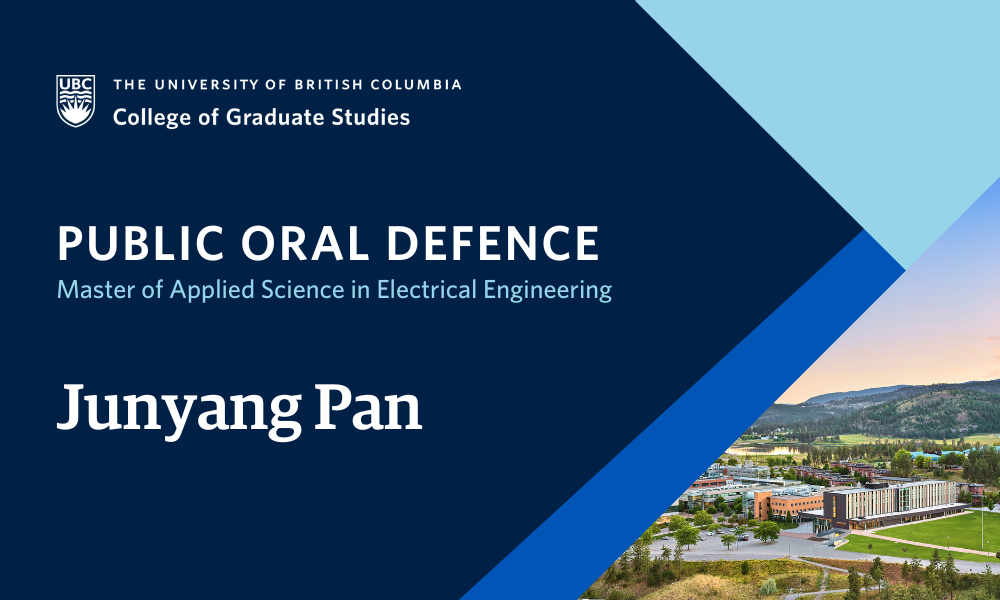
Junyang Pan, supervised by Julian Cheng, will defend their thesis titled “Kolmogorov-Arnold Networks for Spectrum Sensing and Modulation Classification” in partial fulfillment of the requirements for the degree of Master of Applied Science in Electrical Engineering.
An abstract for Junyang Pan’s thesis is included below.
Examinations are open to all members of the campus community as well as the general public. Please email Julian.Cheng@ubc.ca to receive the Zoom link for this exam.
Abstract
In the context of 6G wireless communications and cognitive radio (CR), spectrum sensing and modulation classification are crucial for optimizing spectrum usage and improving communication system performance. Traditional methods, such as energy detection and feature-based techniques, often suffer from reduced accuracy in low signal-to-noise ratio (SNR) environments and are sensitive to noise and interference. While computationally efficient, these methods fall short in complex and dynamic communication settings. Deep learning approaches have been introduced to overcome these limitations, as they can automatically extract features and adapt to changing environments. However, most deep learning models, such as CNNs and LSTMs, come with high computational complexity and large parameter sizes, making them less practical for real-time or resource-constrained applications. Kolmogorov-Arnold Networks (KAN) is a newly proposed architecture, and no practical application studies have been published yet. The authors highlight that KAN has advantages over MLP in terms of both accuracy and interpretability, while also requiring significantly fewer parameters than MLP. To address the limitations of traditional methods and deep learning approaches, this thesis applies KAN to two lightweight solutions: LWSS-KAN for spectrum sensing and SMC-KAN for modulation classification. LWSS-KAN models spectrum sensing as a multi-output binary classification problem, while SMC-KAN models modulation classification as a multi-class classification task using a cross-entropy loss function. By leveraging KAN’s adaptive activation functions, both LWSS-KAN and SMC-KAN achieve high classification accuracy with reduced complexity, especially in high-SNR conditions. Simulation and experimental results show that both models provide accuracy comparable to traditional algorithms, while requiring significantly fewer parameters. The impact of hidden layers and input size in KAN is also explored. These models offer valuable solutions for modern communication systems, particularly in complex environmental scenarios with limited computational resources.