
- This event has passed.
Dissertation Defence: Advanced AI-powered comprehensive framework for modeling, analysis, assessment and performance-based design of ultra-high-performance concrete elements
March 26 at 9:00 am - 1:00 pm
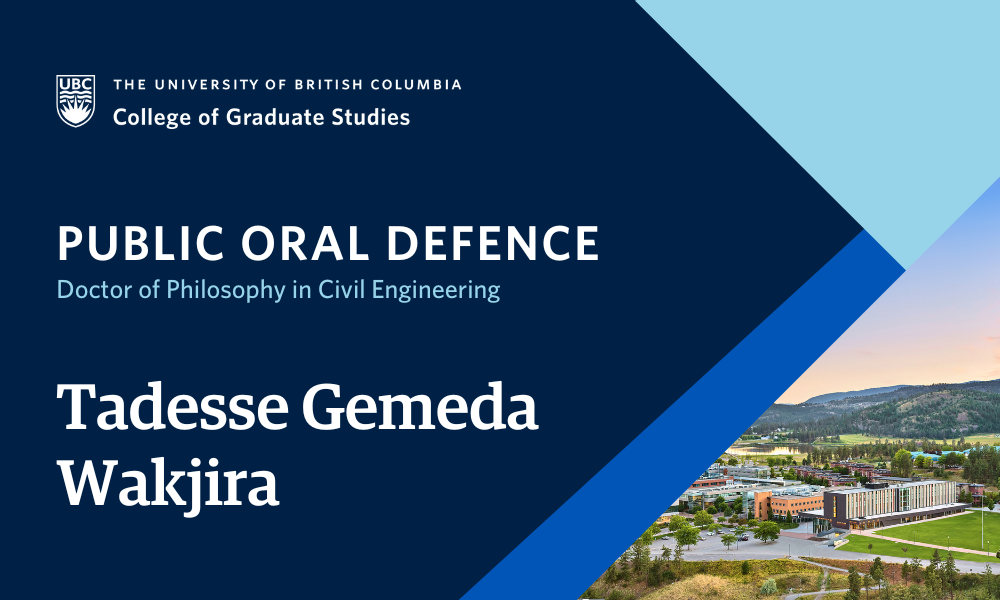
Tadesse Gemeda Wakjira, supervised by Dr. Shahria Alam, will defend their dissertation titled “Advanced AI-powered comprehensive framework for modeling, analysis, assessment and performance-based design of ultra-high-performance concrete elements” in partial fulfillment of the requirements for the degree of Doctor of Philosophy in Civil Engineering.
An abstract for Tadesse Gemeda Wakjira’s dissertation is included below.
Examinations are open to all members of the campus community as well as the general public. Please email shahria.alam@ubc.ca to receive the Zoom link for this defence.
ABSTRACT
This thesis presents an innovative framework for sustainable design, structural modeling, and performance-based seismic design (PBSD) of ultra-high-performance concrete (UHPC) elements utilizing state-of-the-art artificial intelligence techniques. Initially, a novel framework for strength prediction and multi-objective optimization (MOO) of UHPC mixture was introduced using advanced machine learning (ML) and MOO algorithms. A total of 19 objective functions are considered, including cost, uniaxial compressive strength, and 17 environmental impact categories that comprehensively evaluate the environmental sustainability of the UHPC mix. Addressing the need for an accurate stress-strain model of confined UHPC, the thesis introduces a hybrid ML model, augmented by a state-of-the-art conditional tabular generative adversarial network and Optuna to accurately predict the peak and ultimate stress-strain responses of UHPC confined with either normal strength steel (NSS) or high-strength steel (HSS) reinforcement. Furthermore, hybrid ML-based predictive models for complete stress-strain response of UHPC confined with NSS or HSS spirals is proposed. Besides, analytical equations are developed to predict the peak and ultimate stress-strain responses of confined UHPC. Utilizing these models and the uncertainties of optimized UHPC along with other sources of uncertainties, drift capacity limit states are proposed for UHPC columns based on explainable ML models across four different damage states. The efficacy of the developed model is assessed using a range of statistical metrics, including the composite fitness score, which demonstrated a high predictive accuracy of the drift limit states. Moreover, the application of the proposed drift limit states is investigated in the PBSD of UHPC columns. Lastly, the thesis introduces a multivariate seismic fragility assessment approach for UHPC bridges using hybrid ML model.