
Dissertation Defence: Deep neural networks for damage localization in composite materials with ultrasonic guided waves
May 9 at 10:00 am - 2:00 pm
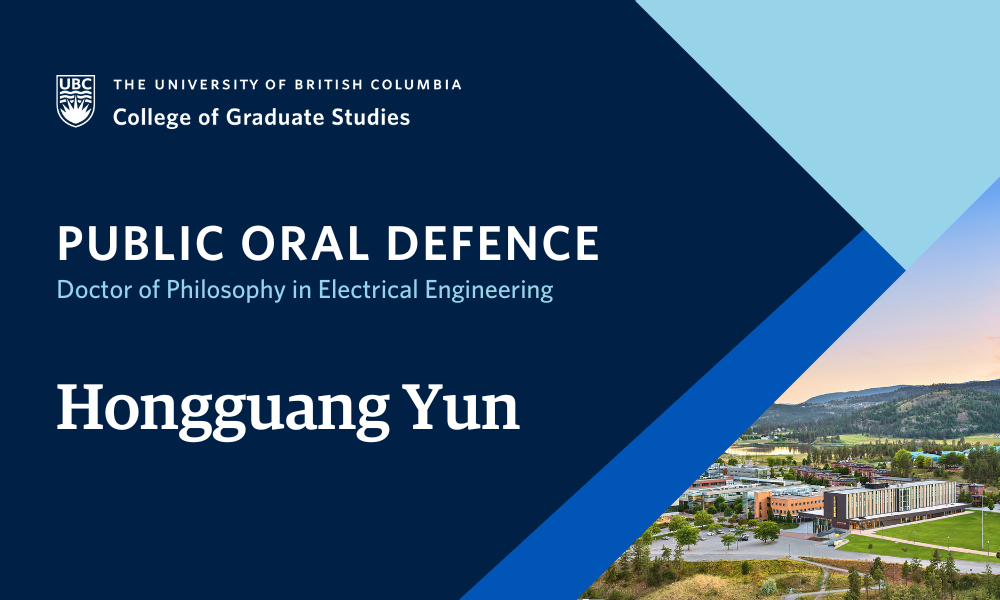
Hongguang Yun, supervised by Dr. Zheng Liu, will defend their dissertation titled “Deep neural networks for damage localization in composite materials with ultrasonic guided waves” in partial fulfillment of the requirements for the degree of Doctor of Philosophy in Electrical Engineering.
An abstract for Hongguang Yun’s dissertation is included below.
Examinations are open to all members of the campus community as well as the general public. Please email zheng.liu@ubc.ca to receive the Zoom link for this defence.
ABSTRACT
Composite materials are widely used in aerospace, wind energy, and shipbuilding, offering significant benefits such as superior strength-to-weight ratios and corrosion resistance. Despite these advantages, they are prone to damage from impacts and environmental stress, necessitating advanced methods for precise damage localization to ensure safety and reduce maintenance costs. Deep learning-based ultrasonic guided wave methods are increasingly recognized for their effectiveness in locating damage in composites. However, many existing studies overlook the distinct requirements between general computer science and industrial applications, often failing to address the challenges of model construction with limited data and the need for embedding physical knowledge to enhance model generalization. This study delves into deep neural network (DNN) models for damage localization, focusing on two main challenges: limited dataset sizes and the integration of physical knowledge into DNN models. It introduces three specific application scenarios—namely, independent and identically distributed ($i.i.d.$) damage localization, domain generalized damage localization, and out-of-distribution (OOD) damage localization—to showcase the DNN model’s potential in addressing various real-life data availability challenges.
Firstly, a multi-dimensional data fusion neural network is developed for $i.i.d.$ damage localization, featuring a novel random Fourier projection head to incorporate low-dimensional data, thereby accelerating model convergence.
Secondly, a domain generalization neural network is proposed, utilizing contrastive learning to enhance the model’s resilience against input variation-induced data distribution discrepancies. This model’s efficacy is validated through its response to varying damage sizes and slight location variations.
Thirdly, a Physics-Informed Neural Network (PINN) model is created for OOD damage localization, drawing inspiration from the analytical ellipse localization method and integrating significant physical constraints, enabling it to accurately locate completely unseen damage during training.
Overall, this study introduces three methodologies that cater to different levels of data availability and physical knowledge, highlighting the complexities of achieving high model performance across varying data distributions. Each method contributes to overcoming the limitations of traditional approaches and improve the robustness and accuracy of damage localization, paving the way for more effective and efficient structural health monitoring in composite materials.