
- This event has passed.
Dissertation Defence: Efficient Online Uncertainty Management: Conformal-In-The-Loop for Measuring and Actioning Uncertainty at Training Time
April 14 at 1:00 pm - 5:00 pm
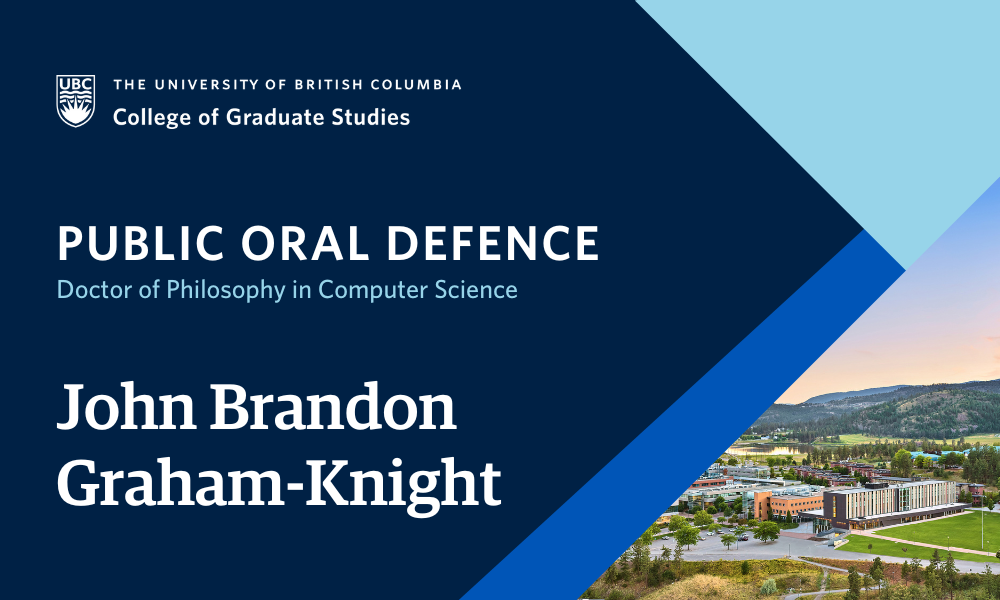
John Brandon Graham-Knight, supervised by Dr. Patricia Lasserre and Dr. Homayoun Najjaran, will defend their dissertation titled “Efficient Online Uncertainty Management: Conformal-In-The-Loop for Measuring and Actioning Uncertainty at Training Time” in partial fulfillment of the requirements for the degree of Doctor of Philosophy in Computer Science.
An abstract for John Brandon Graham-Knight’s dissertation is included below.
Examinations are open to all members of the campus community as well as the general public. This examination will be offered in hybrid format. Registration is not required to attend in person; however, please email patricia.lasserre@ubc.ca to receive the Zoom link for this exam.
ABSTRACT
Focus on Neural Network research has largely been on statistical performance, as Deep Learning is an optimization problem. This often overshadows properties needed for adoption, such as robustness and fairness. Early adversarial attacks showed minor input changes could cause incorrect predictions. Fairness is obscured by the black-box nature of neural networks, which are typically evaluated by a single performance statistic.
This thesis explores uncertainty quantification motivated by improving fairness and robustness. A use case on fair artificial intelligence (AI) explores the Breast Screening Program at the BC Cancer Agency with the evaluation of a commercial AI. Model performance was evaluated using AUC (area under the curve) of ROC (Receiver-operating characteristic) curve methodology, which was consistent with previous studies. Compared to radiologists, it underperforms on follow-up periods up to two years but overperforms for longer periods. It is generalizable across populations, but performs poorly in certain subgroups; significant differences are found for breasts with different BI-RADS densities, patients with a self-reported family history of cancer, and mammograms showing calcifications. A second use case evaluates a car detection algorithm using the KITTI dataset, where the model shows a lack of robustness for small perturbations within sensor error.
These use cases motivate the thesis. Uncertainty is evaluated by comparing the performance and diversity of 144 models from a grid search of network parameters for semantic segmentation of the CityScapes dataset. While many models show no significant performance difference, they show that diversity can reduce variance. This diversity can also be a measure of uncertainty in model ensembles.
Uncertainty quantification during training is often used for online labeling and pruning mislabeled examples. However, these methods are usually computationally expensive and require multiple stages or training runs. The final chapter applies conformal prediction for efficient online data curation in a single training run. It proposes a data-centric method for dynamically weighting examples using prediction set size. Evaluated on CIFAR-10 for classification and CityScapes for segmentation, results show improved accuracy and improved Jaccard Index with small computational overhead.