
- This event has passed.
Fitting Models to Data Not Data to Models Workshop Series: Generalized Additive Models
March 13 at 2:00 pm - 3:00 pm
Free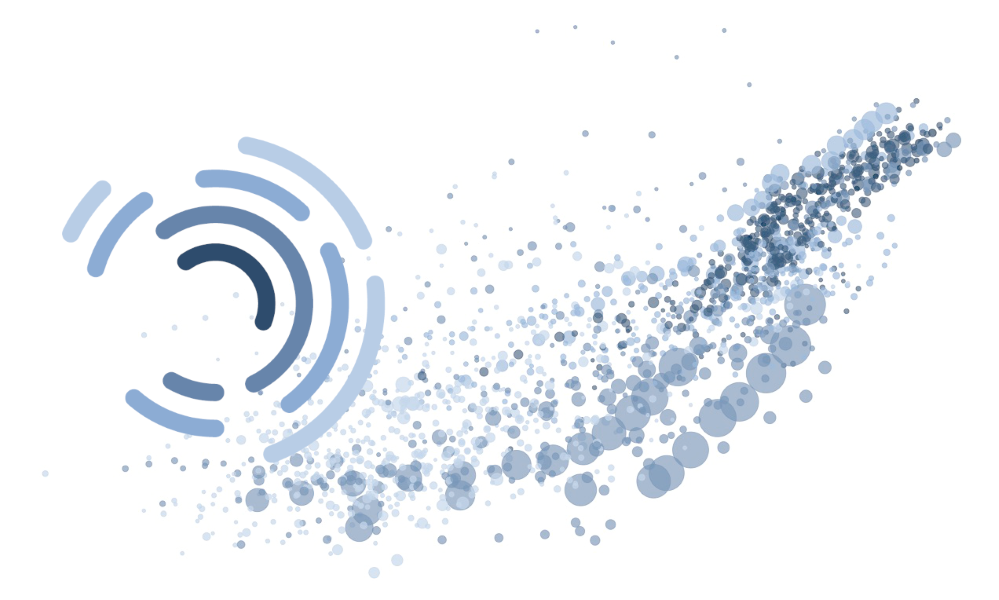
This workshop will introduce Generalized Additive Models (GAMs), which allow one to fit models that are complex and nonlinear but easily interpretable, unlike many “black-box” machine learning models.
By the end of this session, participants will be able to fit GAMs in R
using the mgcv
package and understand the advantages of GAMs over GLMs and LMs.
This workshop qualifies for the Scholarly Research, Writing, and Publishing Credential offered through the College of Graduate Studies.
Fitting Models to Data Not Data to Models Workshop Series
You might have heard someone say that “all models are wrong, but some are useful”. The best way to ensure models are useful is to choose a model that is appropriate to your data and research questions, rather than forcing your data to fit your model’s assumptions (e.g., normality, independence, constant variance).
This series introduces early-career researchers to statistical models that extend beyond linear models (i.e., ANOVAs) so that they may learn how to fit models to their data rather than fitting their data to models. All workshops will use R
and RStudio, so some experience with R
or other programming languages is encouraged but not required. See the R Fundamentals for Data Analysis for an introduction to R
and RStudio. Attendees who do not have experience with R
are encouraged to review this content or take the introductory workshop concurrently if it’s being offered.
You can either register for all workshops, or register for individual workshops that are most applicable to you.