
- This event has passed.
Thesis Defence: Advancing Video Segmentation by Integrating Advanced Learning Paradigms
September 20 at 9:00 am - 1:00 pm
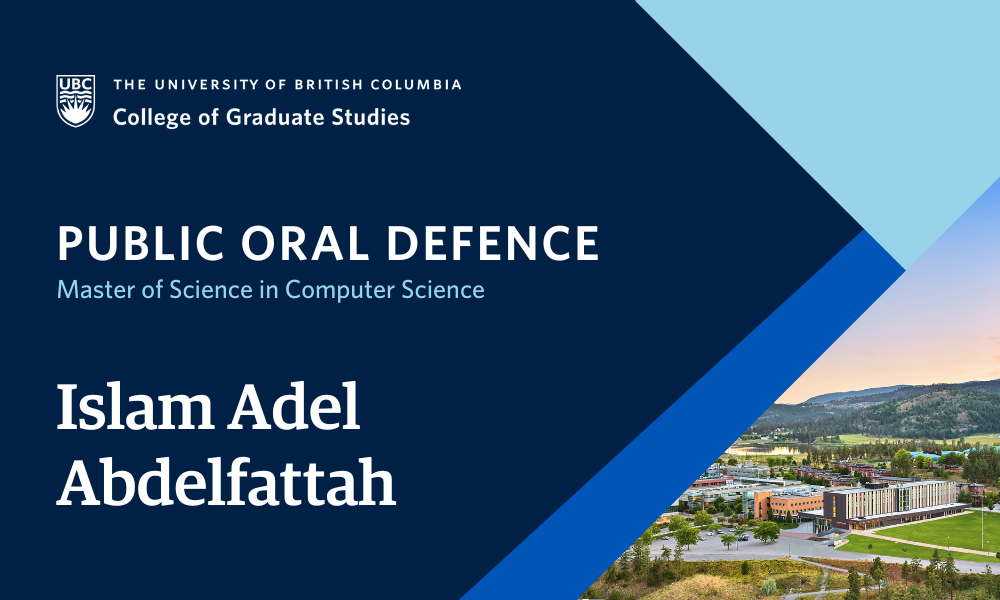
Islam Adel Abdelfattah, supervised by Dr. Mohamed Shehata, will defend their thesis titled “Advancing Video Segmentation by Integrating Advanced Learning Paradigms” in partial fulfillment of the requirements for the degree of Master of Science in Computer Science.
An abstract for Islam Adel Abdelfattah’s thesis is included below.
Defences are open to all members of the campus community as well as the general public. Registration is not required for in person defences.
ABSTRACT
Video segmentation involves isolating specific objects or the foreground from the background in a video, for example, separating people or vehicles from the rest of the scene. This task is crucial in many applications such as video editing, surveillance, autonomous vehicles, and augmented reality. Advanced methods often utilize Deep Learning (DL) to manage the complexities of video sequences, including motion and occlusion. Despite recent progress, current state-of-the-art methods face challenges such as catastrophic forgetting, poor domain generalization, and the loss of object details when objects are occluded by other objects. This thesis addresses these critical challenges in video segmentation by proposing innovative methodologies to enhance segmentation accuracy, robustness, and domain generalization. We introduce a Knowledge Distillation Network (KDNet) and a new training technique to combat catastrophic forgetting and improve domain generalization by leveraging advanced learning paradigms such as federated learning, continual learning, knowledge distillation, and few-shot learning. This approach yields significant improvements across diverse datasets while ensuring data privacy. Additionally, we propose a Mask Enhancement Model (MEM) to mitigate the loss of object details by integrating a foundational model output and employing mask fusion techniques, resulting in better segmentation masks. Experimental results demonstrate KDNet’s ability to achieve state-of-the art domain generalization performance on multiple datasets in foreground segmentation and adapt to new unseen domains using as few as one labelled image (one-shot learning). MEM also proves effective, versatile, and robust performance, achieving state-of-the-art results in Semi-Supervised Video Object Segmentation (S-SVOS).