
Thesis Defence: Applications of Data-Driven Machine Learning for Real-Time Water Quality Monitoring in Water Distribution Systems
April 25 at 9:00 am - 1:00 pm
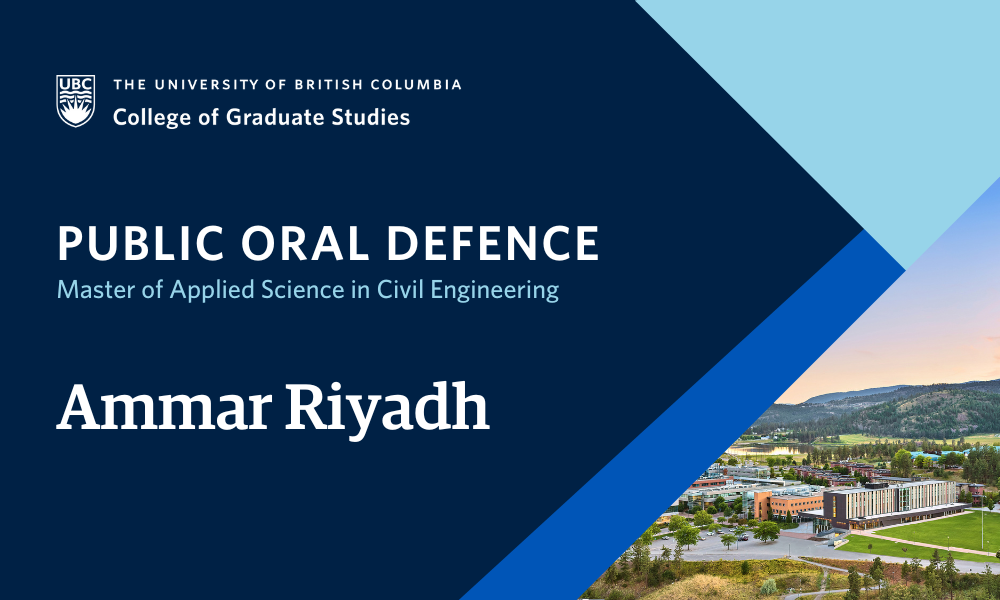
Ammar Riyadh, supervised by Dr. Nicolás Peleato, will defend their thesis titled “Applications of Data-Driven Machine Learning for Real-Time Water Quality Monitoring in Water Distribution Systems” in partial fulfillment of the requirements for the degree of Master of Applied Science in Civil Engineering.
An abstract for Ammar Riyadh’s thesis is included below.
Defences are open to all members of the campus community as well as the general public. Please email nicolas.peleato@ubc.ca to receive the Zoom link for this defence.
Abstract
Chlorine is the most widely used disinfectant globally, essential for maintaining safe drinking water. However, managing chlorine residuals in water distribution systems (WDS) is challenging due to chlorine decay which is influenced by water quality, flow dynamics, and aging infrastructure. Traditional models and infrequent monitoring often lack the accuracy needed for real-time decision-making. To address these limitations, data-driven techniques and real-time monitoring have emerged as promising solutions for optimizing chlorine levels and ensuring regulatory compliance.
This thesis applies machine learning (ML) techniques to improve chlorine residual predictions while enhancing model interpretability. The first study implemented neural networks, including Convolutional Neural Networks (CNN), Long Short-Term Memory (LSTM) networks, and CNN-LSTM architectures, to predict chlorine residuals using historical water quality data. These models outperformed traditional hydraulic models, such as EPANET, achieving mean absolute errors (MAE) below 0.027 mg/L. ML models effectively captured complex non-linear relationships between operational parameters and chlorine decay, demonstrating superior accuracy and robustness.
The second study focused on improving model transparency through Explainable AI (XAI) techniques, including SHapley Additive exPlanations (SHAP), Permutation Feature Importance (PFI), and Occlusion Analysis. These methods validated model logic, identified key input features, and assessed the impact of sensor placement on prediction accuracy. Results indicated that accurate predictions could be achieved with sensors placed far from the prediction site, reducing the need for dense sensor networks. Additionally, sample-specific analysis revealed patterns linking flow rates, temperature, and chlorine decay, aligning with expected water quality behaviors. This enables informed decision-making, such as adjusting operational parameters.
By integrating ML models with XAI techniques, this research supports real-time, data-driven chlorine management, enhancing water quality monitoring while fostering confidence in AI-driven decision-making.