
- This event has passed.
Thesis Defence: Beyond Traditional Single Object Tracking
June 3, 2024 at 11:00 am - 3:00 pm
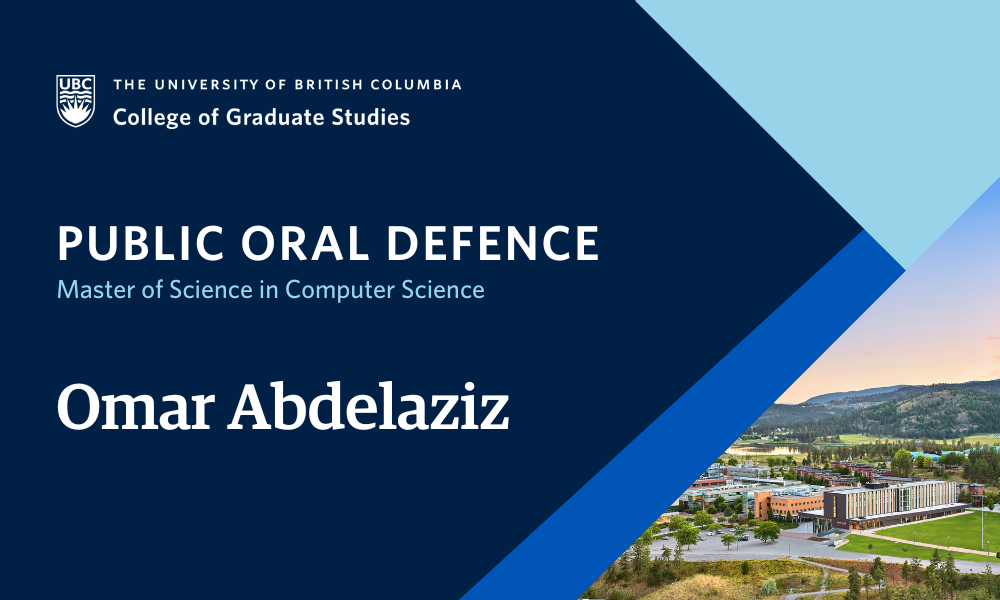
Omar Abdelaziz, supervised by Dr. Mohamed Shehata & Dr. Mohamed Abdelpakey Mohamed, will defend their thesis titled “Beyond Traditional Single Object Tracking” in partial fulfillment of the requirements for the degree of Master of Science in Computer Science.
An abstract for Omar Abdelaziz’s thesis is included below.
Defences are open to all members of the campus community as well as the general public. Registration is not required for in person defences.
ABSTRACT
Single object tracking is a crucial yet challenging task in computer vision. In this task, a model is given the appearance of an arbitrary object in a sequence of frames. The model is required to track the object in all sequence frames. Traditionally, discriminative correlation filters and Siamese convolutional networks have dominated the field but with limitations when tracking challenging objects such as occluded and long-term tracked objects. However, many techniques backed by recent advancements in machine learning theory, such as masked image modelling, generative adversarial networks, variational auto-encoders, and diffusion models, are being increasingly leveraged in single object tracking to provide more customized representations that capture the unique characteristics of the target object.
This thesis explores recent advancements in single object tracking by providing three novel contributions. The first contribution is that we comprehensively survey emerging trends in single object tracking, including sequence models, generative models, and self-supervised learning, proposing a novel categorization for single object tracking methods. We further compare existing approaches and identify promising directions for future research. The second contribution focuses on the critical role of bounding box regression within single object tracking. We argue that leveraging the receptive field of convolutional networks is essential for accurate object localization. We introduce two novel bounding box regression networks that achieve superior performance on benchmark datasets compared to existing methods. The
third contribution addresses the persistent performance gap between training and test data in single object tracking, particularly for transformer-based trackers. It proposes the Deformable Masking Tracker (DMTrack), which injects deformable convolution within the Vision Transformer (ViT) architecture. DMTrack improves the robustness of attentional features, leading to a significant performance boost of up to 2% across seven tracking benchmarks. Overall, this thesis contributes to the field of single object tracking by offering a comprehensive analysis of novel techniques, demonstrating the importance of bounding box regression and proposing a novel solution to bridge the data performance gap. The findings in this thesis pave the way for further advancements in robust and generalizable single object tracking algorithms.