
- This event has passed.
Thesis Defence: Data-Efficient Machine Learning for Predicting Compressive Strength of Fibre-Reinforced Concrete with Waste Rubber and Recycled Aggregate Using Continual and Transfer Learning
June 24, 2024 at 9:00 am - 1:00 pm
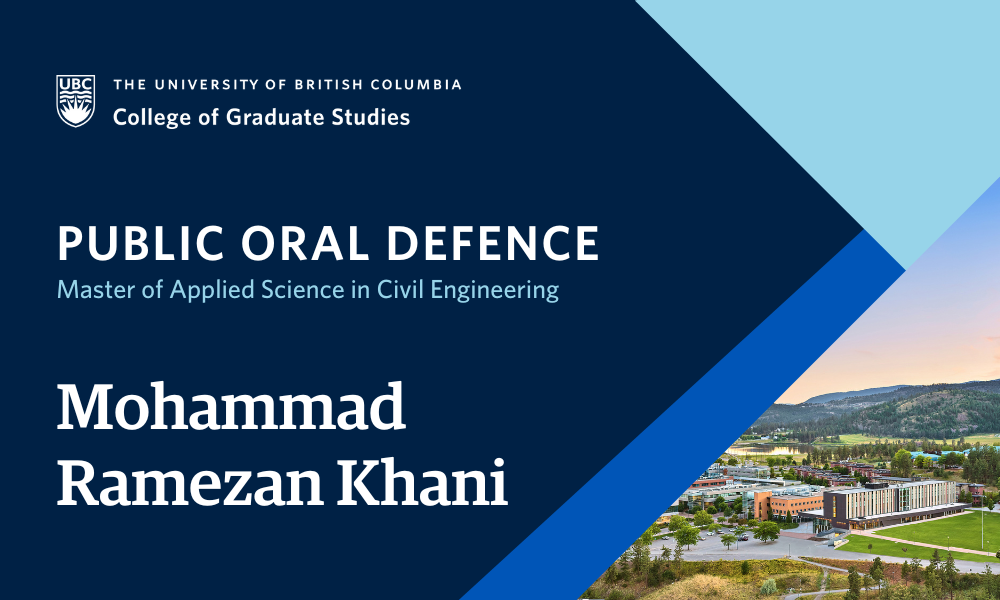
Mohammad Ramezan Khani, supervised by Dr. Shahria Alam, will defend their thesis titled “Data-Efficient Machine Learning for Predicting Compressive Strength of Fibre-Reinforced Concrete with Waste Rubber and Recycled Aggregate Using Continual and Transfer Learning” in partial fulfillment of the requirements for the degree of Master of Applied Science in Civil Engineering.
An abstract for Mohammad Ramezan Khani’s thesis is included below.
Defences are open to all members of the campus community as well as the general public. Please email shahria.alam@ubc.ca to receive the Zoom link for this defence.
ABSTRACT
In recent years, addressing the environmental impact of waste concrete in the construction industry has become increasingly challenging. Additionally, the quantity of discarded tires has significantly increased. Recycled aggregate concrete and crumb rubber have emerged as viable alternatives to natural aggregate in conventional concrete and help preserve natural resources in concrete mixtures. Mining of natural resources has detrimental effects on the environment, primarily due to the associated carbon dioxide emissions. While incorporating both recycled aggregate and crumb rubber in concrete mixtures can help produce greener concrete, these materials negatively impact the concrete’s mechanical properties, especially compressive strength. Extensive engineering tests are essential for determining the mechanical properties of concrete, which are often expensive and time-consuming. To address this challenge, artificial intelligence and machine learning (ML) have been considered as data-driven alternatives. Numerous studies have explored the application of various ML methods in predicting concrete’s mechanical properties. However, these methods have some drawbacks. One major limitation is that the ML models are typically trained for one specific type of concrete and thus not applicable to other types, lacking generalizability. Another issue is the absence of sufficient data for training ML models. In real-world scenarios, the available data for novel kinds of concrete (e.g., fibre-reinforced rubberized recycled aggregate concrete) is limited, which leads to a drastic performance drop in the trained models. This thesis employs a series of data-efficient ML models, namely, continual learning (CL) and transfer learning (TL) to develop a more generalized and adaptable ML framework for concrete mix design applications. Such models leverage the knowledge from related data sources to improve prediction performance in low-data regimes. Two types of TL, namely, domain adaptation and inductive TL are investigated. Additionally, a new CL method is proposed by incorporating two separate neural networks (i.e., main and auxiliary networks) to achieve a more adaptable learning framework applicable to various input space dimensions. The results of TL and CL were compared with classical artificial neural networks, demonstrating that CL and TL models consistently outperform when tested with limited data.