
- This event has passed.
Thesis Defence: Deep Learning Applications in Magnetic Resonance Imaging: Radiofrequency Pulse Design and Automated Real-Time Quality Control
September 17 at 10:00 am - 2:00 pm
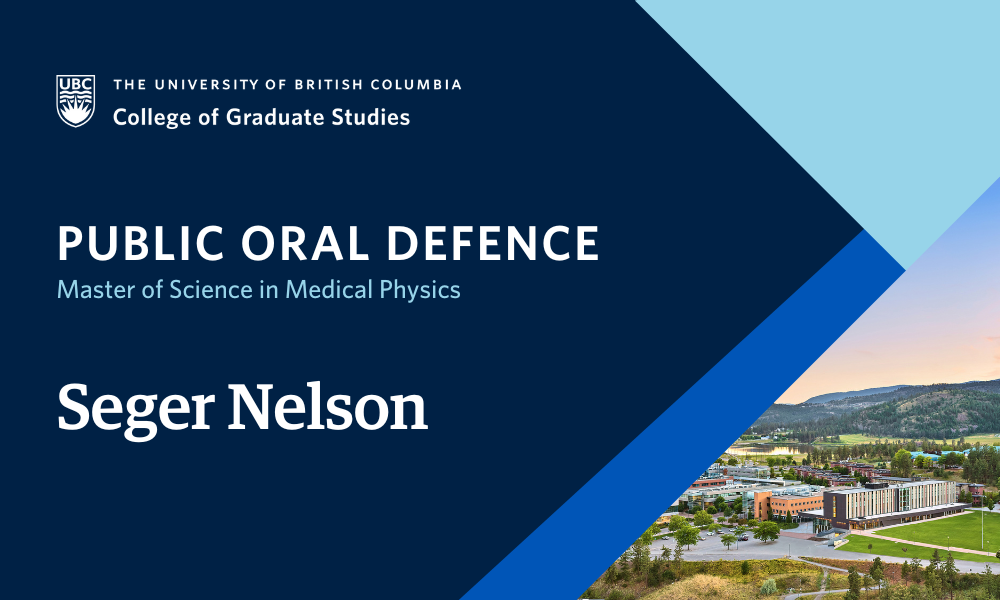
Seger Nelson, supervised by Dr. Rebecca Feldman and Dr. Erin MacMillan, will defend their thesis titled “Deep Learning Applications in Magnetic Resonance Imaging: Radiofrequency Pulse Design and Automated Real-Time Quality Control” in partial fulfillment of the requirements for the degree of Master of Science in Medical Physics.
An abstract for Seger Nelson’s thesis is included below.
Defences are open to all members of the campus community as well as the general public. Registration is not required for in-person defences.
ABSTRACT
Deep Learning–Based Design of RF pulses in MRI
Purpose: The development of custom-designed power-independent-of-number-of-slices (PINS) RF pulses that yield desirable slice profiles requires time-consuming, iterative tuning. The goal of this work was to simplify the design process by training a deep learning (DL) model to generate PINS 180° RF pulses given a desired slice profile.
Methods: A 6-layer feed-forward neural network (NN) was trained with 1.2 Million generated PINS slice profiles and their corresponding 5 pulse characteristic parameter labels. The model predictions were fed into a pulse design algorithm from which an RF pulse and simulated slice profile were generated and used for validation. The root mean squared error of the slice profiles was calculated over the test dataset.
Results: The highest performing model achieved a mean root mean-squarederror (RMSE) of 45.18% between the predicted vs. expected slice profiles in the test dataset.
Conclusion: The model was unable to generalize to unseen data. Future work may entail exploring different DL models, and expanding the train set to include a larger and more diverse set of RF Pulse – Slice Profile pairs.
Towards Deep Transfer Learning-Based Automated Real-Time QC in MRI
Purpose: The frequent use of RF coils in MRI occasionally causes hardware failures. Often, failures may not be visually recognizable, and are only caught during routine quality control (QC) procedures. Images acquired between failures and QC checks may possess a reduction in diagnostic quality. This can lead to costly patient re-scans that can negatively impact wait times, diagnosis, and treatment planning. This problem was investigated by training a DL model on a labeled dataset of passed and failed MRI images to recognize a coil failure.
Methods: Patient images from instances of passed and failed RF coils were collected by referencing the Interior Health Authority QC logsheet to create labeled datasets. Three pre-trained Convolutional Neural Networks (CNNs) associated with image classification were trained to differentiate
between images taken with passed and failed RF coils. receiver operating characteristic (ROC) curves and accuracy were used to evaluate the model on a test dataset.
Results: The highest performing model achieved an accuracy of 0.73 when evaluated on the test set.
Conclusion: These results serve as a proof of concept for deep transfer learning–based RF coil failure recognition in MRI images. However, the models show signs of overfitting and further investigation is needed.