
- This event has passed.
Thesis Defence: Exploring the Integration of Deep Learning into the Photogrammetric Pipeline
December 8, 2023 at 9:00 am - 1:00 pm
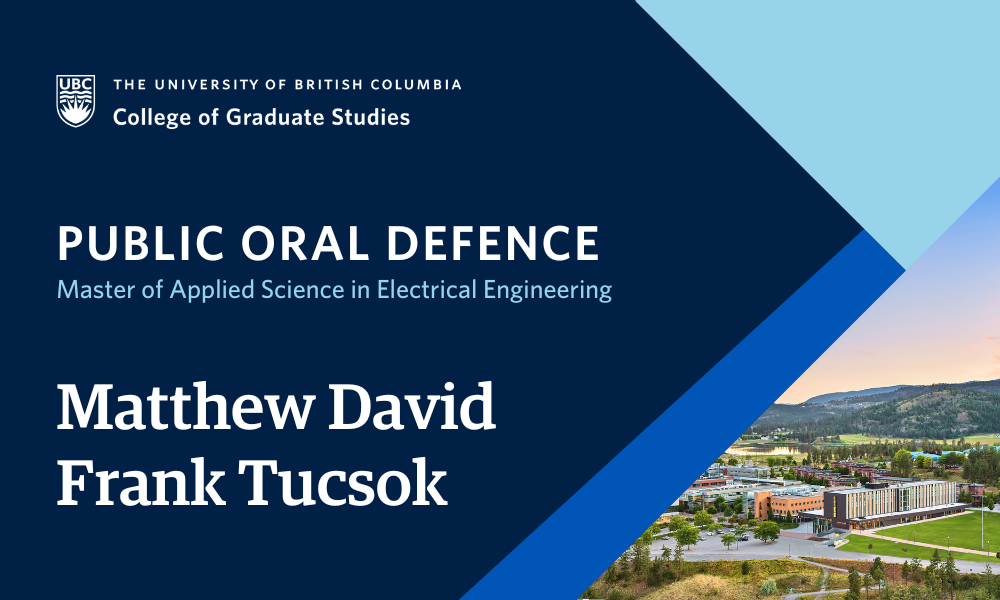
Matthew David Frank Tucsok, supervised by Dr. Homayoun Najjaran & Dr. Sumi Siddiqua, will defend their thesis titled “Exploring the Integration of Deep Learning into the Photogrammetric Pipeline” in partial fulfillment of the requirements for the degree of Master of Applied Science in Electrical Engineering.
An abstract for Matthew David Frank Tucsok’s thesis is included below.
Defences are open to all members of the campus community as well as the general public. Please email sumi.siddiqua@ubc.ca to receive the Zoom link for this defence.
ABSTRACT
This thesis explores the integration of deep learning into the photogrammetric pipeline through the introduction of deep learning into both the algorithms at the core of photogrammetry and through camera guidance during data acquisition to improve reconstruction performance. The first of these explorations highlights the difference in reconstruction performance between traditional feature extraction and matching techniques versus a deep feature matcher in an ideal simulated environment and custom Structure-from-Motion (SfM) pipeline. The results of this exploration highlights the need for a utility metric to quantify which views are more important for 3D reconstruction. This is accomplished by sort-
ing views based on the reconstruction performance of a deep learning-based Single-view AutoEncoder(SAE) trained on 3D reconstruction from single views. These sorted views indicate the utility of a view,
which then can be used for improving downstream reconstruction tasks.
To test the utility of these sorted views, a novel deep learning-based model is proposed, which fuses a graph neural network originally designed for camera relocalization with an Long Short-Term Memory (LSTM) layer for sequential capture processing. This fusion of architectures allows the model to learn both spatial and temporal relationships between views via training on an augmented view dataset which leverages the utility metric provided by the SAE. The model, called the Graph-Best-View-Finder (GBVF), provides an end-to-end solution which given a sequence of RGB images iteratively constructs a graph embedding of the 3D scene in order to suggest the pose of the next best view. Alongside GBVF development, a comprehensive package named the View Planning Toolbox is developed to automate view planning dataset generation, trajectory visualization, and reconstruction coverage evaluation.