
- This event has passed.
Thesis Defence: Robust Optimization for Plastic Closed-Loop Supply Chains: Advancing Data-Driven Models With Kernel Weight Adjustment And Fairness Metrics
November 14, 2024 at 11:00 am - 3:00 pm
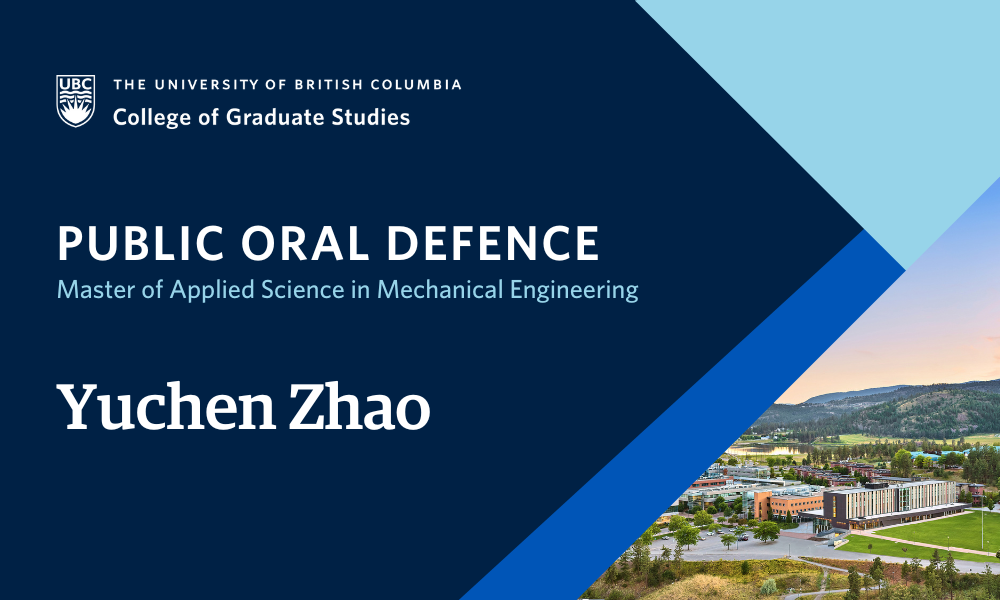
Yuchen Zhao, supervised by Dr. Babak Mohamadpour Tosarkani, will defend their thesis titled “Robust Optimization for Plastic Closed-Loop Supply Chains: Advancing Data-Driven Models With Kernel Weight Adjustment And Fairness Metrics” in partial fulfillment of the requirements for the degree of Master of Applied Science in Mechanical Engineering.
An abstract for Yuchen Zhao’s thesis is included below.
Defences are open to all members of the campus community as well as the general public. Please email babak.tosarkani@ubc.ca to receive the Zoom link for this defence.
ABSTRACT
Recycling is essential for reducing the environmental impact of plastic waste and is a key part of sustainable supply chains. However, optimizing these networks is challenging due to uncertainties in input parameters like demand and return rates, requiring solutions to enhance efficiency and resilience in Closed-Loop Supply Chains (CLSC). This research focuses on designing robust CLSC networks to improve resource efficiency, reduce costs, and maximize recycling rates, addressing the need for more resilient and sustainable systems. This research develops a novel approach using Data-Driven Robust Optimization (DDRO), which incorporates historical data into the optimization process to effectively deal with uncertainties such as fluctuating demand and variable return rates of recycled materials.
A key innovation in this research is the introduction of a novel concept in DDRO approaches, focusing on the construction of balanced uncertainty sets. A ”balanced uncertainty set” is defined as one where the boundaries maintain nearly equal distances in all directions to the uncovered data samples, ensuring a more equitable representation of uncertainty. To achieve balanced uncertainty sets across varying conservatism levels, a single-Kernel learning-based approach is developed, wherein the weights of the basis kernel matrices are adjusted based on the data’ dispersion in each direction. Additionally, this thesis introduces a Robust Fairness (RF) index to evaluate and compare the balance of different uncertainty sets. A data-driven algorithm is further developed to efficiently compute the RF index. Numerical results demonstrate that the proposed DDRO approach in this thesis generates more balanced uncertainty sets compared to competing methods. In addition, the proposed data-driven algorithm can effectively compute the RF index and compare uncertainty sets in terms of balance, without introducing computational complexities.