
- This event has passed.
Thesis Defence: Smart Dynamic Trajectory Planning of Autonomous Underwater Vehicles Using Reinforcement Learning and Historical Data
October 25 at 2:00 pm - 6:00 pm
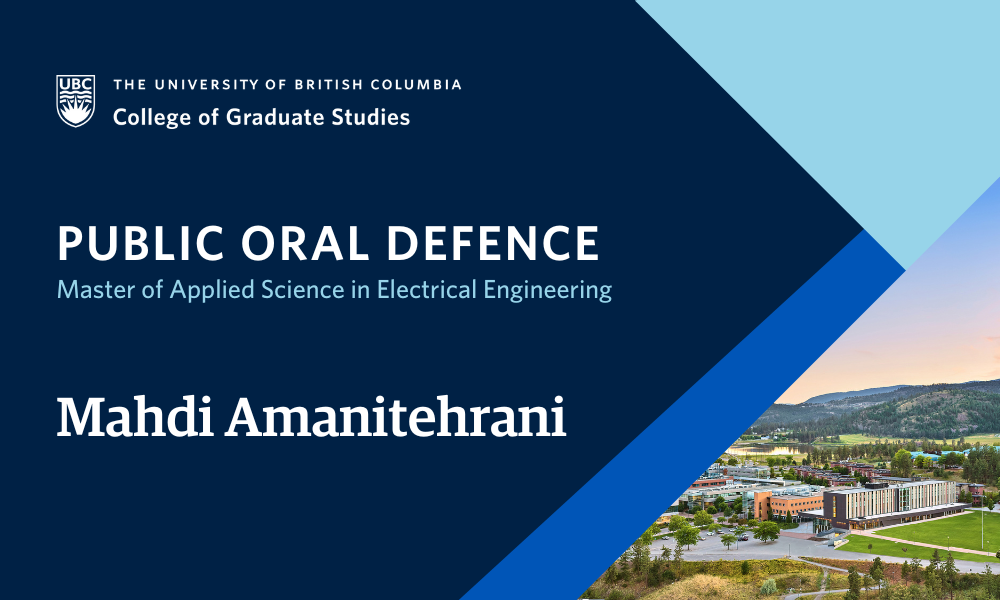
Mahdi Amanitehrani, supervised by Dr. Julian Cheng, will defend their thesis titled “Smart Dynamic Trajectory Planning of Autonomous Underwater Vehicles Using Reinforcement Learning and Historical Data” in partial fulfillment of the requirements for the degree of Master of Applied Science in Electrical Engineering.
An abstract for Mahdi Amanitehrani’s thesis is included below.
Examinations are open to all members of the campus community as well as the general public. Please email Julian.Cheng@ubc.ca to receive the Zoom link for this exam.
Abstract
Underwater wireless sensors are essential in ocean studies and applications, providing continuous monitoring and recording of critical data. Their remote operation over extended periods requires energy-efficient designs, and one major challenge is collecting data from these sensors, as long-range communication increases energy consumption. Furthermore, unlike terrestrial GPS, the lack of reliable localization underwater means the exact locations of these sensors are initially unknown. Autonomous Underwater Vehicles (AUVs) play a crucial role in detecting and retrieving data from these sensors. To achieve this, intelligent AUVs must dynamically plan their trajectories based on current detection information, aiming to collect as much data as possible from wireless networks. In this thesis, we first mathematically examine the AUV dynamic trajectory planning problem. Recognizing the inherent challenges, we propose methods to improve upon existing approaches by incorporating historical AUV data into real-time decision-making. Two trajectory planning methods are introduced, with the second utilizing Deep Reinforcement Learning (DRL) to optimize path planning. Simulation results show promising improvements over prior methods, significantly narrowing the gap between dynamic trajectory planning and the upper bounds of ideal methods under strong assumptions.