
- This event has passed.
Thesis Defence: The Effectiveness of GNNs for Node Classification: The Significance of Side Information
May 17, 2024 at 9:00 am - 1:00 pm
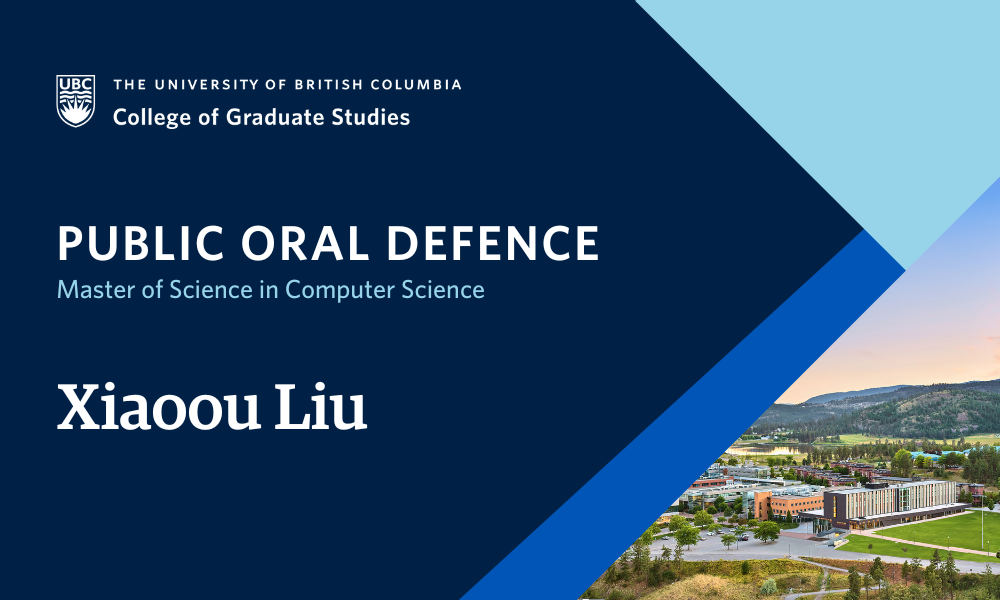
Xiaoou Liu, supervised by Dr. Yong Gao, will defend their thesis titled “The Effectiveness of GNNs for Node Classification: The Significance of Side Information” in partial fulfillment of the requirements for the degree of Master of Science in Computer Science.
An abstract for Xiaoou Liu’s thesis is included below.
Defences are open to all members of the campus community as well as the general public. Please email yong.gao@ubc.ca to receive the Zoom link for this defence.
ABSTRACT
This thesis studies the effectiveness of Graph Neural Networks (GNNs) for node classification. We conduct systematic experiments on several representative deep-learning models for graph data, using training data generated from the Stochastic Block Model (SBM) and the theoretical results on the fundamental limits of this model as guidance in the design of our experiments. While GNNs are widely believed to be powerful learning models for graph data, our empirical findings suggest that they do not necessarily outperform other machine-learning-based methods and traditional algorithms for node classification. In particular, we observe that GNN-based methods fail to exploit the information from labelled nodes in semi-supervised learning settings. We propose an effective technique to enhance GNN-based methods by making better use of labelled information in the training data. Our experiments using synthetic data from SBMs and real-world datasets demonstrate that our method can significantly enhance the capabilities of GNN models and notably improve their performance for node classification. Additionally, in the context of unsupervised learning, we discuss the possibility of incorporating other types of side information into GNNs that may exist in multiplex network data.