
- This event has passed.
Thesis Defence: Unsupervised Learning Approach for Detection, Discovery, and Automatic Labeling of Unseen Faults
October 8, 2024 at 11:00 am - 3:00 pm
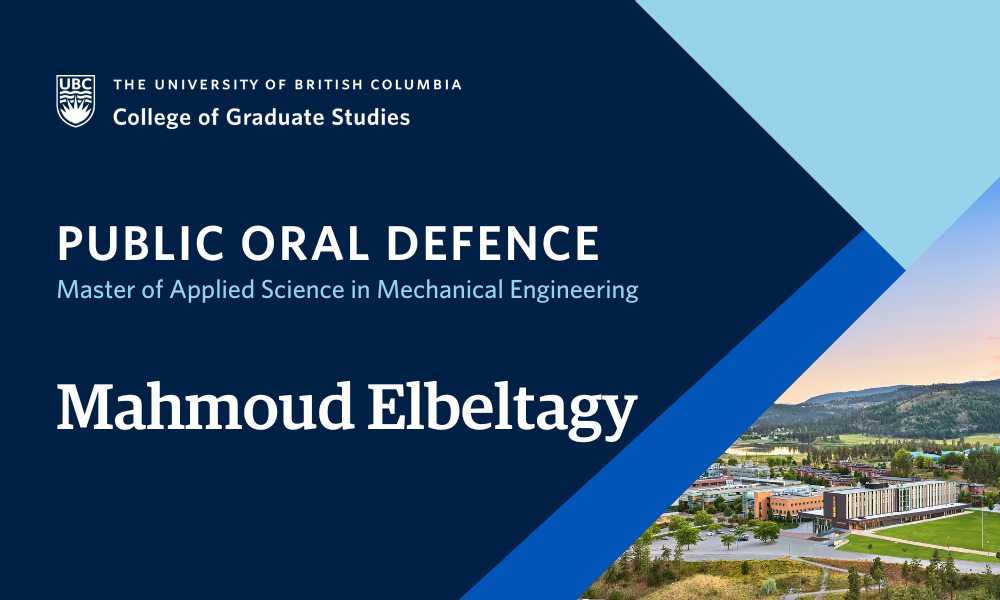
Mahmoud Elbeltagy, supervised by Dr. Ahmad Al-Dabbagh, will defend their thesis titled “Unsupervised Learning Approach for Detection, Discovery, and Automatic Labeling of Unseen Faults” in partial fulfillment of the requirements for the degree of Master of Applied Science in Mechanical Engineering.
An abstract for Mahmoud Elbeltagy’s thesis is included below.
Defences are open to all members of the campus community as well as the general public. Please email ahmad.aldabbagh@ubc.ca to receive the Zoom link for this defence.
ABSTRACT
Fault diagnosis is crucial in industrial process operation. Addressing the fault by taking the right course of action is crucial for ensuring efficient and safe operation of a facility. To that end, fault classification algorithms are superior to fault detection algorithms. However, they require the existence of a labeled dataset or extensive knowledge of the process, which is not realistic in real-world scenarios. Thus, this work presents a fault discovery system that differentiates unknown faults while relying only on data from normal operating conditions. In addition, it performs fault localization to describe each discovered fault. To that end, an autoencoder, which consists of a linear state-space-like Probabilistic Recurrent Neural Network and a Convolutional Neural Network, is used for fault detection and feature extraction. Fault types are then separated by clustering the extracted features using the Density-Based Spatial Clustering of Applications with Noise algorithm. The feature extraction is performed so that the correlation information between the process variables and the extracted features is easily attained. This facilitates describing different faults by determining the process variables that are most associated with these faults. In the end, a dataset with virtual labels and a fault localization rule describing the discovered faults are reported. Field experts can then inspect the generated set to label the discovered faults and design procedures for addressing them in the future.