
- This event has passed.
Thesis Defence: Extraction of Social Determinants of Health from Electronic Health Records using Natural Language Processing
December 13, 2023 at 2:00 pm - 6:00 pm
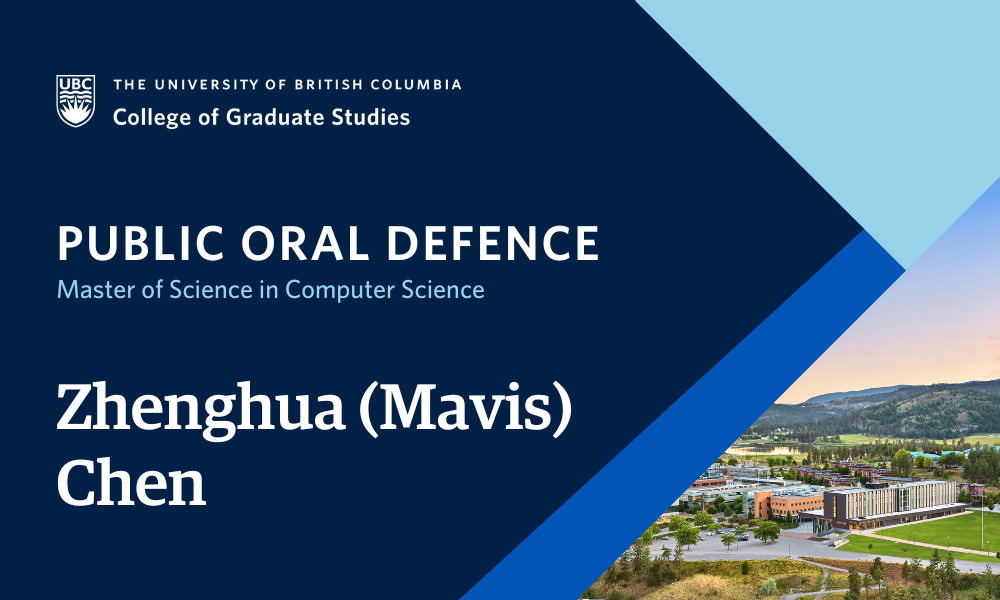
Zhenghua (Mavis) Chen, supervised by Dr. Rasika Rajapakshe and Dr. Patricia Lasserre, will defend their thesis titled “Extraction of Social Determinants of Health from Electronic Health Records using Natural Language Processing” in partial fulfillment of the requirements for the degree of Master of Science in Computer Science.
An abstract for Zhenghua (Mavis) Chen’s thesis is included below.
Defences are open to all members of the campus community as well as the general public. Registration is not required for in person defences.
ABSTRACT
Purpose: Social Determinants of Health (SDoH) have a significant impact on human health outcomes and disparities. Collecting SDoH from electronic health records can facilitate decision-making and downstream research. With thousands of clinical records, automated extraction methods using Artificial Intelligence (AI) would be more efficient and cost-effective. This study aims to autonomously extract comprehensive SDoH details from Electronic Health Records (EHR) using a Natural Language Processing (NLP) based AI pipeline.
Methods: One thousand documents from BC Cancer with concentrated SDoH information were carefully selected and labeled to provide the ground truth for training and assessing the NLP models. Two pipelines were applied for SDoH extraction: an open source pipeline trained on the BC Cancer dataset and an industrial pre-trained solution used as a benchmark. To optimize the performance of the first pipeline, three experiments were conducted to justify the effect of including subtype word positions during training on the extraction performance. The results of two pipelines were compared and the best performing one was subsequently employed for the extraction of SDoH information from a total of 13,258 oncology documents.
Results: The open-source pipeline gained an average F1 score of 0.88 on the validation dataset for extracting 13 SDoH factors, outperforming the benchmark by 5%. This pipeline also demonstrated a notably superior capability to extract detailed subtypes compared with the benchmark. The benchmark was advantageous in identifying rarely documented SDoH types in the data for extraction in this work. As a result, 60,717 SDoH factors and associated details were extracted from the oncology documents from the BC Cancer EHR. The most frequently extracted SDoH are Tobacco Use, Employment Status, Marital Status, Alcohol Consumption, and Living Status, which occurred from 8k to 12k times.
Conclusion: The NLP pipeline successfully extracted a wide array of SDoH factors from clinical notes, achieving commendable performance despite being trained on a relatively small labeled dataset.