
- This event has passed.
Thesis Defence: Using Wasserstein Distance and Conditionality for Stable and Selective Data Augmentation in Pavement Crack Analysis
September 10, 2024 at 11:00 am - 3:00 pm
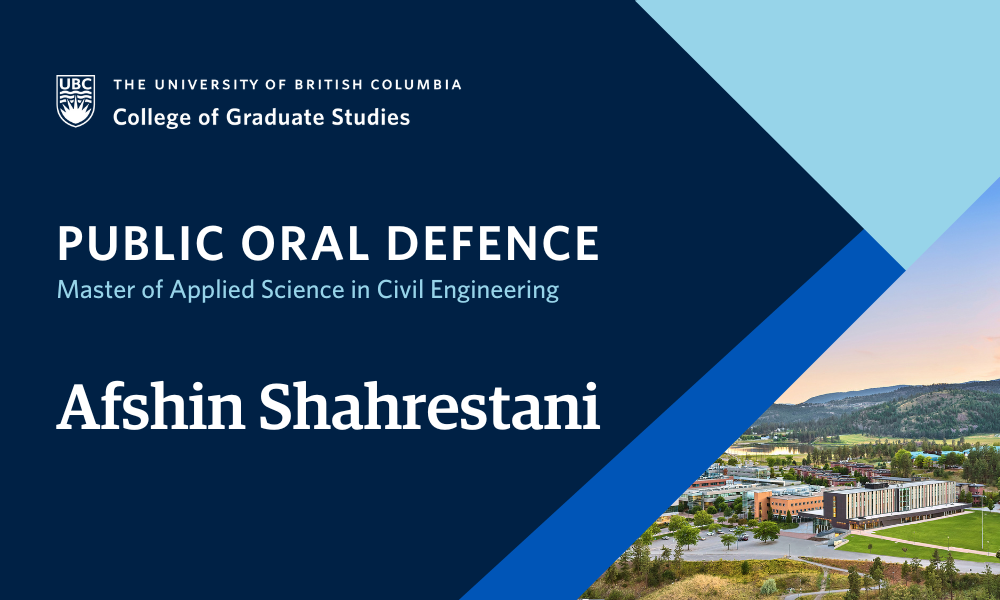
Afshin Shahrestani, supervised by Dr. Suliman Gargoum, will defend their thesis titled “Using Wasserstein Distance and Conditionality for Stable and Selective Data Augmentation in Pavement Crack Analysis” in partial fulfillment of the requirements for the degree of Master of Applied Science in Civil Engineering.
An abstract for Afshin Shahrestani’s thesis is included below.
Defences are open to all members of the campus community as well as the general public. Please email suliman.gargoum@ubc.ca to receive the Zoom link for this defence.
ABSTRACT
Pavement crack detection and treatment is one of the vital tasks in road infrastructure management. Failure to do so in a proactive manner increases maintenance costs and results in a drop in the structural integrity of the pavement. Deep learning techniques hold substantial promise for automating the detection of pavement cracks in highway maintenance facilitating proactive management of such defects. As a result, such techniques can reduce the financial cost, and time and remove the human bias in the pavement defect analysis process. One barrier to large-scale adoption of deep learning techniques in this area is the scarcity of high-quality, and balanced training datasets. Inherent data imbalances, where certain crack types predominate over others, lead to detection bias and overfitting in machine learning models. This is exacerbated by variations in crack types, skewing the model training towards more frequently occurring cracks, thereby diminishing efficacy in identifying less-common types of cracks. Data augmentation is one of the possible solutions to these issues.
Mode Collapse, training instability, and lack of agency in data generation were identified as the main challenges associated with previous pavement crack augmentation methods. To overcome the challenges, the thesis introduces two Generative Adversarial Network (GAN) architectures: Wasserstein GAN (WGAN), and Conditional WGAN (C-WGAN). These models aim to remedy the shortcomings of previous data augmentation methods used in pavement crack analysis.
The research utilizes annotated images from the Crack500 and CrackForest datasets, categorized into transverse, longitudinal, block, and alligator crack types. These images are used as the training data for both the GANs and a baseline classifier, aimed at measuring the impact of synthetic crack images in augmented datasets. The findings demonstrate the effectiveness of WGAN and C-WGAN in addressing the limitations of previous augmentation methods, generating high-quality, diverse synthetic images. The GAN-augmented models achieved an average classification score improvement of 5\% over the baseline. While WGAN generated slightly higher-quality images, C-WGAN offered the advantage of user-specific image generation. Both models proved to be effective data augmentation tools for pavement crack datasets, each with distinct advantages and potential trade-offs. This research contributes to the field by providing robust GAN-based solutions for enhancing pavement crack detection and classification.